Fixing the research-to-policy pipeline
How researchers can help strengthen confidence in evidence
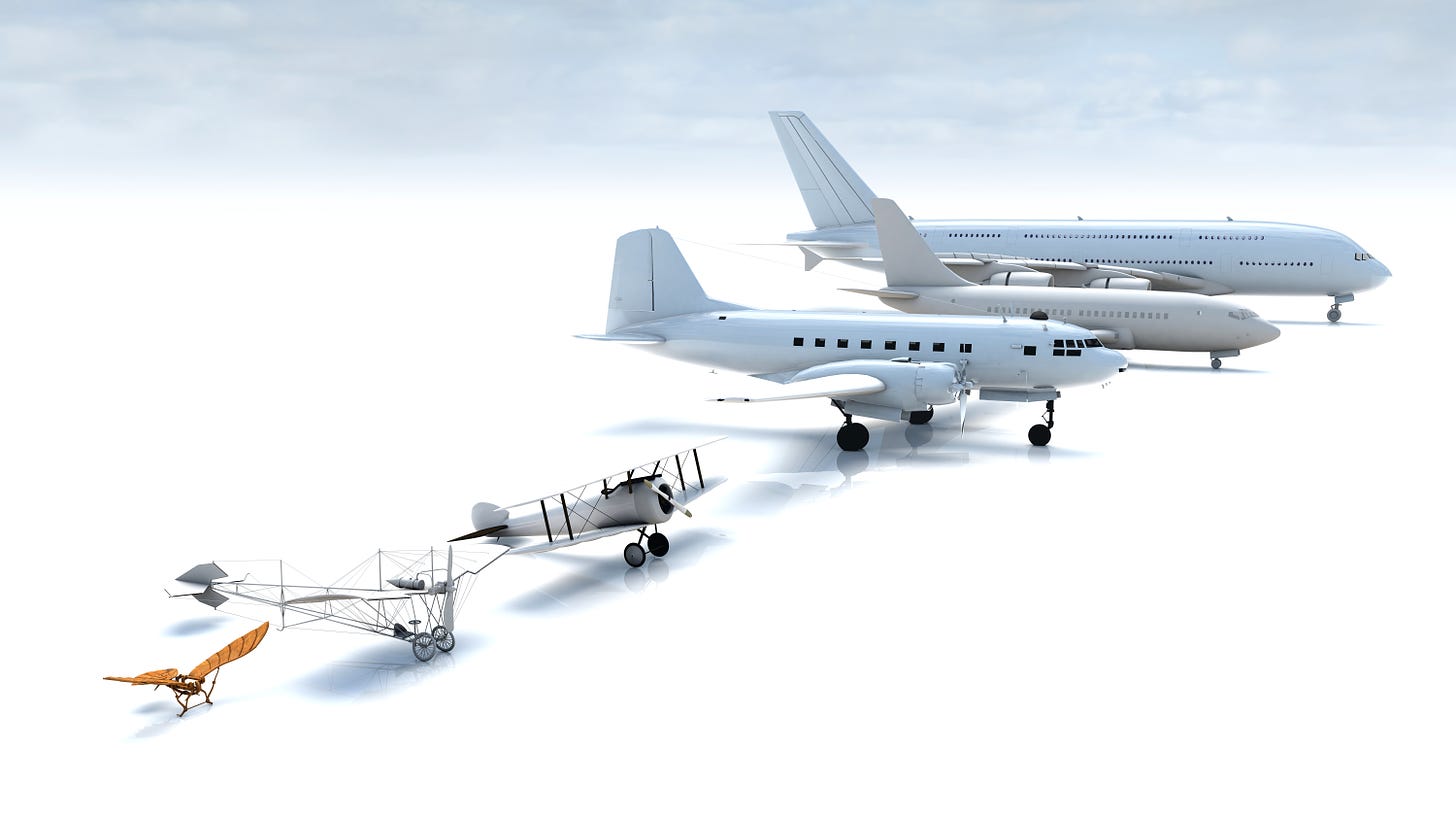
Those of us dedicated to achieving evidence-based policy face many challenges. Convincing practitioners to engage in rigorous evaluations of their programs and policies is a big one. Convincing elected leaders to care about the evidence is another. But in between these challenges is the challenge of interpreting the evidence that has been produced, to determine which approaches to label “evidence-based.”
In her recent essay, “Cause, Effect, and the Structure of the Social World,” law professor Megan Stevenson highlights several ways our current evidence-production systems can lead us astray. The naïve approach she criticizes goes something like this: Run a randomized controlled trial. If results are statistically significant in the hoped-for direction, recommend the program as evidence-based policy.
As Stevenson notes, there are at least four ways published research results could be misleading, if evidence-based policy is our goal. First, a statistically significant result could be due to luck. Second, it could be due to p-hacking (defined below). Third, results might have limited external validity. Fourth, scaling effective programs is often difficult.
For much of her essay, Stevenson lumps these challenges together as collective evidence that published research results don’t tell us much about whether a program is effective or should be scaled. Separating these challenges leads to a much more optimistic conclusion than Stevenson reached.
First, we have luck. Journal referees and editors have a notorious preference for statistically significant results. That is, given two studies with the same research question and statistical power, they will be more likely to publish the one that found statistically significant evidence that a program worked (or didn’t). Editors and referees don’t like null results. However, using conventional levels of statistical significance (a p-value less than 0.05, which means that there’s a less than 5% chance that the null hypothesis is true), we must face the statistical reality that, if the true causal relationship between a given intervention and a given result is zero (that is, a null effect), one out of 20 studies of that relationship will produce a false positive. That is, for every 19 studies showing a null effect, we should expect to see one that shows a statistically significant effect. If that last study is the only one that gets published, then published results are misleading.
The primary way to address this challenge is one that is now quite widespread: pre-registration of all RCTs conducted. Pre-registration means that researchers create a public record that a study is planned, as well as some basic information about it. Many research funders (including Arnold Ventures) now require this, as does the American Economic Association (if you want the option to publish your study in one of its prestigious journals). This ensures we have a record of all studies that were conducted, before they got underway. If we later see that 19 of the 20 RCTs of some program were never published, we should be suspicious that the program had no impact. Over time, pre-registration should give us confidence that published results — without evidence of a deep “file drawer” of null results — represent a true effect.
We are now in the enviable position where we have a variety of evidence-based solutions that we would like to scale. But scaling is easier said than done.
Next, we have p-hacking. This is when researchers adjust the regressions they run, or the outcomes they consider, to find one that produces a significant finding. It is a direct result of the publication bias described above: Researchers know their odds of publication are much higher if they have a statistically significant result, so they find a way to produce a statistically significant result. (And, as above, the more regressions you run, the more likely you are to find a false positive.)
There are three main approaches to addressing this challenge. One is requiring pre-analysis plans, which force researchers to pre-commit to (at least) the main outcome of interest. This keeps them from fishing for significant results when they have a long list of potential outcome measures. Second is requiring a lengthy appendix of robustness checks — as is now the norm in economics. The spirit of these checks is to show that, for every judgment call the researchers made, they should show how the results change if they made different choices. Third is requiring that researchers upload their data and code when they publish their papers, so that other researchers can check for themselves how sensitive the results are to minor changes in the specification. These approaches are not as widespread as they should be. For instance, most criminology journals do not require researchers to share their data or code for replication purposes, and long appendices of robustness checks are rare outside of economics. But, when implemented, these approaches make it much more difficult for researchers to p-hack their way to a significant result.
Third, we have external validity. In this case, a result is internally valid — it measures the true causal effect of a program as it was implemented in a particular place — but it turns out, something about that context was key to the program’s efficacy, and the program would not be effective elsewhere. For instance, a study might find that air conditioning in a Texas prison dramatically reduces violent incidents. The effects of the same intervention — air conditioning — might be different in Minnesota prisons, because the summers in Minnesota are not as hot.
This simple example points to the solution: Do more studies to help us understand the effects in different contexts. Sometimes the reasons for the differences will be obvious; in my simple example, local temperatures surely make air conditioning more or less important. But sometimes it will take us a lot of digging to figure out when an intervention is effective, and for whom. This doesn’t mean building a valid evidence base isn’t possible, it just means it is hard. If we’re serious about addressing important social problems, we can’t shy away from hard work.
Finally, we have challenges of scaling. Even after we convince ourselves that a program would be effective in lots of places, we need to figure out whether we can actually reproduce it in all those other places — and if so, how to do so. There are a variety of reasons this is difficult. Some of them have to do with logistics — who is in charge of setting up all these new programs, and how clear is the guidance we are giving them about how those programs should work (to reproduce the original effects)? How confident are we that, even with clear guidance, the programs will be implemented as designed? Other challenges relate to resource constraints. For instance, a program that serves 100 people might employ 10 people, but a program that serves 100,000 people would need to employ 10,000 people. Are 10,000 people with sufficient qualifications available to do this work? Will they be as good at the job as the first 10 that were hired? (Probably not.)
This is the challenge for which solutions are least developed. Contrary to Stevens’ original claim, we are now in the enviable position where we have a variety of evidence-based solutions that we would like to scale. But scaling is easier said than done. Going forward, social scientists would be wise to invest in a “science of scaling” to figure out how to scale effective programs. As an example, a study by Jonathan Davis and colleagues suggests that, when researchers need to hire 50 people to staff a program, they should recruit 1,000, rank them, and then hire 50 at random. This clever approach would help researchers test how much less effective the 900th person hired might be than the first person hired. We need a lot more research like this — and for more studies to incorporate the approaches they develop — to improve our success in taking effective programs to scale.
The four challenges to evidence-based policy that Stevenson identifies are not insurmountable obstacles. They are valid concerns, to be sure, but the first three have been top of mind for the research community for years, and we have made substantial progress in addressing them. Finding solutions to difficult problems is what researchers do best. Let’s keep going.
Jennifer Doleac is executive vice president of criminal justice at Arnold Ventures. She is an economist who specializes in the economics of crime and criminal justice policy. Arnold Ventures is a financial supporter of the Niskanen Center.